Facility type and volume impact outcomes in acute myeloid leukemia—a National Cancer Database Study
Highlight box
Key findings
• Academic centers and high volume facilities had better OS, and a greater likelihood of treatment with chemotherapy and allogeneic HSCT.
• Black race and Hispanic ethnicity were associated with differences in TTT, OS, and receipt of different therapies, with largely worse outcomes.
What is known and what is new?
• Treatment facility characteristics impact outcomes, and resource rich facilities have greater experience with managing treatment related complications, and access to novel treatment options like clinical trials.
• Shorter TTT, observed at low volume facilities, did not necessarily lead to improved clinical outcomes. Palliative care was more likely at low volume compared to high volume facilities.
What is the implication, and what should change now?
• Differences exist in outcomes in AML patients based on treatment facility type, volume, and racial/ethnic background. It is imperative to increase access at multiple levels to ensure equitable care for all AML patients.
Introduction
Acute myeloid leukemia (AML) affects older patients (1) and is associated with a poor prognosis with a 5-year survival of approximately 30% (2). Demographic and socioeconomic factors impact treatment utilization and outcomes of AML patients (3-5). Prior studies have demonstrated that lower socioeconomic status, female sex, uninsured or Medicare insurance status, older age, comorbidities, being unmarried, Black race, and Hispanic ethnicity are linked to decreased utility of chemotherapy and hematopoietic stem cell transplant (HSCT) (5-10). Lower overall survival (OS) has been associated with Black race, being a Medicaid beneficiary, uninsured, single, divorced, less educated, and residing in a county within the lower 3 quintiles of median household income (4,11-16). Additionally, treatment facility characteristics are known to impact survival. Resource rich facilities have more favorable patient outcomes, like short-term mortality rates (17-20). The purpose of our study using the National Cancer Database (NCDB) was to determine whether characteristics of the treating facility affect outcomes. Our primary objective was to assess how facility type and volume impact time to treatment (TTT). Secondary objectives examined the impact of these hospital characteristics on OS and receipt of chemotherapy, allogeneic HSCT and/or palliative care. We hypothesized that academic centers and high-volume facilities have shorter TTT, better OS, and higher utilization rates of induction chemotherapy, allogeneic HSCT, and palliative care. We present this article in accordance with the STROBE reporting checklist (available at https://ace.amegroups.com/article/view/10.21037/ace-22-12/rc).
Methods
Data source and patient selection
This was a retrospective cross-sectional study of hospital-based data from the NCDB, a joint project of the Commission on Cancer (CoC) of the American College of Surgeons and the American Cancer Society. The study was exempt from review by the Georgetown University Medical Center Institutional Review Board as the dataset was existing, publicly available, and de-identified. The study was conducted in accordance with the Declaration of Helsinki (as revised in 2013). Using NCDB participant user file data from reporting facilities on five hematologic malignancies (AML, acute lymphocytic leukemia, chronic lymphocytic leukemia, chronic myeloid leukemia, and multiple myeloma), from 453,027 patients, we identified 124,988 with AML over the age of 18 (Figure 1). Data available at the time of our study was a convenience sample of hospitalized patients diagnosed from January 1, 2004 to December 31, 2016. International Classification of Diseases for Oncology version 3 codes 9840–9861, 9865–9874, 9891–9931 captured patients of interest and included patients with acute monocytic leukemia and AML but excluded acute promyelocytic leukemia. For the evaluation of our outcomes of interest, missing data were excluded as outlined in Figure 1.
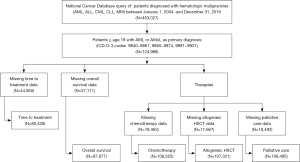
Variables
The variables abstracted included facility characteristic data (facility type and hospital volume), sex, age, race, Hispanic origin, education (adults without a high school diploma), distance traveled for therapy (great circle distance), urban/rural location of reporting facility, insurance type (including Medicaid expansion status state group), and Charlson/Deyo score (21). The Charlson/Deyo score was a weighted score (0 to ≥3), computed from the sum of scores of different comorbidities. Myocardial infarction, congestive heart failure, peripheral vascular disease, cerebrovascular disease, dementia, chronic pulmonary disease, rheumatologic disease, peptic ulcer disease, mild liver disease, and diabetes each were assigned a score of 1. A score of 2 was assigned to diabetes with chronic complications, hemiplegia/paraplegia, and renal disease, a score of 3 for moderate or severe liver disease, and finally a score of 6 for acquired immunodeficiency syndrome (AIDS). Education was derived from area-based metrics (zip code) rather than being patient specific. Urban/rural classification was defined using the typology published by the USDA Economic Research Service. Metropolitan counties were determined by population size, and nonmetropolitan counties by degree of urbanization and adjacency to metropolitan areas.
Facility types were defined by the CoC, based on the number of newly diagnosed cases/year. Community cancer programs had 100–500 newly diagnosed cancer cases/year, comprehensive community cancer programs ≥500 newly diagnosed cancer cases/year, academic centers >500 newly diagnosed cancer cases/year and post-graduate medical education, and integrated network cancer programs were multiple facilities that came together to provide integrated cancer care to treat a variable number of newly diagnosed cancer cases/year. Using the distribution of cases reported by each facility over the period, high volume facilities were defined as those with case volumes in the top 1 percentile (greater than or equal to the 99th percentile), as in prior NCDB work (22). All other facilities were classified as low volume facilities. In our study, the cutoff for high volume facilities corresponded to those treating ≥662 patients.
Outcomes
The primary outcome was TTT, and secondary outcomes were OS and receipt of chemotherapy, allogeneic HSCT, and/or palliative care. Chemotherapy was defined as systemic and cytotoxic antileukemia drugs. Palliative care was defined as any care provided to alleviate symptoms without curative intent and included systemic therapy (chemotherapy, immunotherapy), and/or pain management therapy. TTT was defined as the number of days between the date of diagnosis and the date on which any treatment (systemic, curative, or palliative intent) began at any facility. OS was based on the number of days, weeks, or months from diagnosis to last contact/death and vital status at last contact (alive or deceased).
Statistical analysis
Time-to-event endpoints including TTT, and OS were compared across different facility types and volumes using the Log-rank test and Kaplan-Meier Method. For the multivariable adjusted analyses, a Cox proportional hazard model was used adjusting for demographic and socioeconomic confounding variables listed above in the variables section. These confounding variables were considered to ensure internal validity of our study and give appropriate estimates of the association between our exposure and outcome variables. Binary outcomes including receipt of chemotherapy, allogeneic HSCT, and/or palliative care were assessed with logistic regression using unadjusted and adjusted models with the same confounders. We performed additional subgroup analyses, and evaluated our outcome of interest with respect to the number of CoC facilities where care was received, age (<60 and ≥60), and academic center volume (high versus low). We also did a time dependent analyses, and the year of diagnosis was used for creation of subgroups. Odds ratios (ORs) and hazard ratios (HRs) were presented with 95% confidence intervals (CIs). A two-sided P≤0.05 was considered statistically significant. No multiple test adjustment was applied. All statistical analysis was done using R (version 4.0.3).
Results
Baseline characteristics
There were 124,988 AML patients with a median age of 63 years (range, 18–90 years). There were 68,043 (54%) males, 107,363 (86%) White and 110,712 (89%) non-Hispanic patients. The median age of diagnosis was earliest among Hispanic Whites at 55 years, followed by Hispanic Blacks at 57 years, non-Hispanic Blacks at 60 years, and latest for non-Hispanic Whites at 67 years. There were 54,961 patients (44%) treated at academic centers, and 98,983 (79%) patients received treatment at low volume facilities. A Charlson/Deyo score of 0 or no comorbid conditions was found in 88,553 (71%) patients. Additionally, 101,039 (81%) patients lived in metro counties, and 35,606 (28%) lived in areas where 6.3–10.8% of adults did not graduate from high school. The most common type of insurance was Medicare possessed by 62,076 patients, 50% of the cohort. There were 95,345 (76%) patients who received chemotherapy. Palliative care and allogeneic HSCT were offered to 4,111 (3%) and 5,651 (5%) patients respectively (P<0.001 for all, Table 1).
Table 1
Variables | Values | P value |
---|---|---|
Age at diagnosis (years), median [range] | 63 [18–90] | |
Sex, n (%) | <0.001 | |
Male | 68,043 (54.0) | |
Female | 56,945 (46.0) | |
Race, n (%)† | <0.001 | |
White | 107,363 (86.0) | |
Black/African American | 10,877 (9.0) | |
Native Hawaiian and other Pacific Islander | 929 (0.7) | |
American Indian and Alaska Native | 415 (0.3) | |
Asian | 2824 (2.0) | |
Other | 1,088 (0.9) | |
Unknown | 1,492 (1.1) | |
Hispanic origin, n (%) | <0.001 | |
Non-Hispanic | 110,712 (89.0) | |
Hispanic | 7,841 (6.0) | |
Unknown | 6,435 (5.0) | |
No high school diploma, n (%) | <0.001 | |
<6.3% | 30,873 (25.0) | |
6.3–10.8% | 35,606 (28.0) | |
10.9–17.5% | 31,550 (25.0) | |
≥17.6% | 25,186 (20.0) | |
Unknown | 1,773 (2.0) | |
Urban/rural residence, n (%)‡ | ||
Metro counties | 101,039 (81.0) | <0.001 |
Urban counties | 18,320 (14.0) | |
Rural counties | 2,343 (2.0) | |
Unknown | 3,286 (3.0) | |
Charlson/Deyo score, n (%) | <0.001 | |
0 | 88,553 (71.0) | |
1 | 24,579 (20.0) | |
2 | 7,930 (6.0) | |
≥3 | 3,926 (3.0) | |
Great circle distance (miles), median [range] | 46 [0–5,077] | <0.001 |
Facility type, n (%) | <0.001 | |
Academic Center | 54,961 (44.0) | |
Comprehensive Community Cancer Program | 37,757 (30.0) | |
Integrated Network Cancer Program | 12,840 (10.0) | |
Community Cancer Program | 6,351 (5.0) | |
Unknown | 13,079 (11.0) | |
Facility case volume, 99th percentile cutoff [range] | 662 [1–1,819] | <0.001 |
Facility volume, n (%) | <0.001 | |
Low volume | 98,983 (79.0) | |
High volume | 26,005 (21.0) | |
Insurance status, n (%) | <0.001 | |
Medicare | 62,076 (50.0) | |
Private insurance | 44,111 (35.0) | |
Medicaid | 9,315 (8.0) | |
Uninsured | 4,050 (3.0) | |
Unknown | 3,856 (3.0) | |
Other government | 1,580 (1.0) | |
Medicaid expansion status state group, n (%)§ | <0.001 | |
Non-expansion state | 42,607(34.0) | |
January 2014 expansion state | 34,721 (28.0) | |
Early expansion state (2010–2013) | 18,900 (15.0) | |
Late expansion state (after January 2014) | 15,681 (13.0) | |
Suppressed (age <40) | 13,079 (10.0) | |
Treatments, n (%) | <0.001 | |
Chemotherapy | 95,345(76.0) | |
Allogeneic hematopoietic stem cell transplant | 5,651 (5.0) | |
Palliative care | 4,111 (3.0) | |
Other¶ | 2,638 (2.0) | |
Unknown | 17,243 (14.0) |
†, race categorizations based on US Census Bureau. ‡, urban/rural categorization: Metro counties: counties in metro areas with populations of 250,000–1,000,000; Urban counties: urban population of 2,500–20,000 both adjacent and non-adjacent to a metro area; Rural counties: completely rural or <2,500 urban population both adjacent and non-adjacent to a metro area. §, Medicaid Expansion Status State Group: Non-expansion states: Tennessee, North Carolina, Idaho, Georgia, Florida, Missouri, Alabama, Mississippi, Kansas, Texas, Wisconsin, Utah, South Carolina, South Dakota, Virginia, Oklahoma, Nebraska, Wyoming, Maine; Jan 2014 expansion states: Kentucky, Nevada, Colorado, Oregon, New Mexico, West Virginia, Arizona, Rhode Island, Maryland, Massachusetts, North Dakota, Ohio, Iowa, Illinois, Vermont, Hawaii, New York, Delaware; Early expansion states (2010–2013): Washington, California, New Jersey, Minnesota, District of Columbia, Connecticut; Late expansion states (after Jan 2014): New Hampshire, Indiana, Michigan, Pennsylvania, Arkansas, Montana, Louisiana. ¶, other treatments included immunotherapy and autologous transplant. Treatments were not mutually exclusive.
TTT
Median TTT was longest at community cancer programs at 7 days (95% CI: 6–7 days), and shortest at integrated network cancer programs at 4 days (95% CI: 4–4 days) (P<0.0001, Figure 2A). This was consistent in our multivariable adjusted analysis, with all facilities having shorter TTT than community cancer programs (academic centers: HR 1.17, 95% CI: 1.13–1.22; comprehensive community cancer programs: HR 1.21, 95% CI: 1.17–1.26; integrated network cancer programs: HR 1.29, 95% CI: 1.24–1.34) (P<0.001 for all, Table 2). For volume, similar median TTT was noted at 5 days for high volume facilities (95% CI: 5–5) and 4 days (95% CI: 4–4 days) at low volume facilities (P<0.0001, Figure 2B). However, after adjusting for demographic and socioeconomic factors, the multivariable analysis found low volume facilities had statistically significant shorter TTT than high volume facilities (HR 1.05, 95% CI: 1.04–1.07, P<0.001, Table 2).
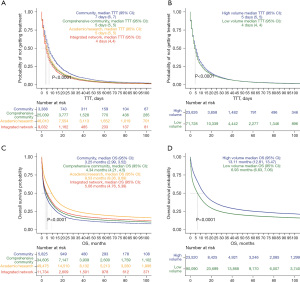
Table 2
Variable | Time to treatment | |
---|---|---|
HR, 95% CI | P value | |
Facility type | ||
Comprehensive Community versus Community | 1.21, 1.17–1.26 | <0.001 |
Academic versus Community | 1.17, 1.13–1.22 | <0.001 |
Integrated Network versus Community | 1.29, 1.24–1.34 | <0.001 |
Facility volume | ||
Low versus high volume | 1.05, 1.04–1.07 | <0.001 |
Sex | ||
Female versus male | 1.03, 1.02–1.05 | <0.001 |
Age | 0.99, 0.99–0.99 | <0.001 |
Race | ||
Black/African American versus White | 0.95, 0.93–0.97 | <0.001 |
Native Hawaiian and other Pacific Islander versus White | 1.09, 1.00–1.18 | 0.049 |
American Indian and Alaska Native versus White | 0.89, 0.79–1.00 | 0.052 |
Asian versus White | 1.04, 0.99–1.10 | 0.087 |
Others versus White | 0.94, 0.87–1.02 | 0.130 |
Unknown versus White | 1.03, 0.96–1.11 | 0.350 |
Hispanic origin | ||
Hispanic versus non-Hispanic | 0.95, 0.92–0.98 | 0.035 |
Unknown versus non-Hispanic | 1.04, 1.00–1.07 | 0.031 |
No high school diploma | ||
10.9–17.5% versus ≥17.6% | 0.98, 0.96–1.01 | 0.150 |
6.3–10.8% versus ≥17.6% | 1.01, 0.99–1.04 | 0.180 |
<6.3% versus ≥17.6% | 1.01, 0.99–1.04 | 0.220 |
Urban/rural | ||
Urban versus metro | 1.09, 1.07–1.11 | <0.001 |
Rural versus metro | 1.14, 1.09–1.20 | <0.001 |
Charlson/Deyo score | ||
1 versus 0 | 1.12, 1.10–1.14 | <0.001 |
2 versus 0 | 1.13, 1.10–1.17 | <0.001 |
≥3 versus 0 | 1.10, 1.05–1.14 | <0.001 |
Great circle distance | 0.9998, 0.9998–0.9999 | <0.001 |
HR, hazard ratio; CI, confidence interval.
Other factors that influenced TTT included race, ethnicity, and county of residence. Among Blacks (HR 0.95, 95% CI: 0.93–0.97, P<0.001, Table 2) and Hispanics (HR 0.95, 95% CI: 0.92–0.98, P=0.035, Table 2), TTT was longer when compared with Whites and non-Hispanics respectively. Shorter TTT was seen with residence in urban (HR 1.09, 95% CI: 1.07–1.11, P<0.001, Table 2) and rural (HR 1.14, 95% CI: 1.09–1.20, P<0.001, Table 2) counties compared to metro counties.
There were no major differences in TTT based on number of CoC programs where care was received (=1 versus >1), age (<60 versus ≥60), and academic center volume (high versus low volume). Additionally, there were no major differences noted in a time dependent analyses from 2004–2007, 2008–2011, and 2012–2016.
OS
Median OS was the longest at academic centers at 9.53 months (95% CI: 9.36–9.69 months), and shortest at community cancer programs at 3.25 months (95% CI: 2.99–3.52 months) (P<0.0001, Figure 2C). The multivariable analysis demonstrated improvement in OS only at academic centers when compared to all facility types (HR 0.90, 95% CI: 0.87–0.93, P<0.001, Table 3). High volume facilities had better median OS than low volume facilities [13.11 months (95% CI: 12.81–13.47 months); 6.93 months (95% CI: 6.83–7.06 months), P<0.0001, Figure 2D]. This was consistent in the multivariable analyses with worse OS at low volume facilities (HR 1.14, 95% CI: 1.12–1.16, P<0.001, Table 3).
Table 3
Variable | Overall survival | |
---|---|---|
HR, 95% CI | P value | |
Facility type | ||
Comprehensive Community versus Community | 1.01, 0.98–1.04 | 0.530 |
Academic versus Community | 0.90, 0.87–0.93 | <0.001 |
Integrated Network versus Community | 0.99, 0.96–1.03 | 0.670 |
Facility volume | ||
Low versus high volume | 1.14, 1.12–1.16 | <0.001 |
Sex | ||
Female versus male | 0.97, 0.96–0.98 | <0.001 |
Age | 1.05, 1.04–1.05 | <0.001 |
Race | ||
Black/African American versus White | 1.06, 1.04–1.09 | <0.001 |
Native Hawaiian and other Pacific Islander versus White | 0.90, 0.82–0.99 | 0.036 |
American Indian and Alaska Native versus White | 1.01, 0.89–1.16 | 0.860 |
Asian versus White | 0.88, 0.84–0.93 | <0.001 |
Others versus White | 0.83, 0.75–0.91 | <0.001 |
Unknown versus White | 1.04, 0.97–1.11 | 0.300 |
Hispanic origin | ||
Hispanic versus non-Hispanic | 0.86, 0.83–0.89 | <0.001 |
Unknown versus non-Hispanic | 1.06, 1.03–1.09 | 0.019 |
No high school diploma | ||
10.9–17.5% versus ≥17.6% | 0.97, 0.95–1.00 | 0.019 |
6.3–10.8% versus ≥17.6% | 0.94, 0.92–0.96 | <0.001 |
<6.3% versus ≥17.6% | 0.86, 0.85–0.88 | <0.001 |
Urban/rural | ||
Urban versus metro | 1.01, 0.99–1.03 | 0.180 |
Rural versus metro | 1.04, 0.99–1.10 | 0.093 |
Charlson/Deyo score | ||
1 versus 0 | 1.23, 1.21–1.25 | <0.001 |
2 versus 0 | 1.47, 1.43–1.51 | <0.001 |
≥3 versus 0 | 1.72, 1.66–1.79 | <0.001 |
Great circle distance | 0.9999, 0.9998–0.9999 | <0.001 |
HR, hazard ratio; CI, confidence interval.
Race, ethnicity, Charlson/Deyo score, education and female sex impacted OS. Blacks had worse OS (HR 1.06, 95% CI: 1.04–1.09, P<0.001, Table 3). A Charlson/Deyo score of ≥3 was associated with the worst OS (score of 1 HR of 1.23, 95% CI: 1.21–1.25; score of 2 HR of 1.47, 95% CI: 1.43–1.51; score of ≥3 HR of 1.72, 95% CI: 1.66–1.79, P<0.001 for all, Table 3). Residence in areas with the greatest levels of education (HR 0.86, 95% CI: 0.85–0.88), Hispanic ethnicity (HR 0.86, 95% CI: 0.83–0.89), and female sex (HR 0.97, 95% CI: 0.96–0.98) improved OS (P<0.001, Table 3).
In patients treated at >1 CoC program, OS was worse at integrated network cancer programs (HR 1.17, 95% CI: 1.07–1.29, P<0.001). Among patients <60 years, better OS was noted at academic centers and integrated network cancer programs (academic centers HR 0.89, 95% CI: 0.82–0.97, P<0.001; integrated network cancer programs HR 0.90, 95% CI: 0.81–0.98, P<0.022). No major differences were noted in OS in the time dependent analyses from 2004–2007, 2008–2011, and 2012–2016.
Receipt of chemotherapy, allogeneic HSCT, and/or palliative care
Academic centers had 2.44 (95% CI: 2.29–2.60, P<0.001) and 5.56 (95% CI: 4.05–7.64, P<0.001) higher odds of chemotherapy and allogeneic HSCT respectively (Table 4). Patients at low volume facilities had 1.25 higher odds of palliative care (95% CI: 1.12–1.40, P<0.001, Table 4) and a lower likelihood of receiving both chemotherapy and allogeneic HSCT (chemotherapy OR 0.41, 95% CI: 0.39–0.44, P<0.001; allogeneic HSCT OR 0.60, 95% CI: 0.55–0.64, P<0.001; Table 4).
Table 4
Variables | Chemotherapy | Allogeneic hematopoietic stem cell transplant | Palliative care | |||||
---|---|---|---|---|---|---|---|---|
OR, 95% CI | P value | OR, 95% CI | P value | OR, 95% CI | P value | |||
Facility type | ||||||||
Comprehensive Community versus Community | 1.47, 1.38–1.56 | <0.001 | 2.29, 1.66–3.16 | <0.001 | 0.98, 0.86–1.11 | 0.780 | ||
Academic versus Community | 2.44, 2.29–2.60 | <0.001 | 5.56, 4.05–7.64 | <0.001 | 0.91, 0.8–1.04 | 0.190 | ||
Integrated Network versus Community | 1.71, 1.59–1.84 | <0.001 | 3.83, 2.75–5.32 | <0.001 | 1.11, 0.96–1.28 | 0.160 | ||
Facility volume | ||||||||
Low versus high volume | 0.41, 0.39–0.44 | <0.001 | 0.60, 0.55–0.64 | <0.001 | 1.25, 1.12–1.40 | <0.001 | ||
Sex | ||||||||
Female versus male | 0.90, 0.87–0.92 | <0.001 | 1.01, 0.95–1.07 | 0.810 | 1.06, 0.99–1.13 | 0.071 | ||
Age | 0.92, 0.92–0.92 | <0.001 | 0.93, 0.93–0.93 | <0.001 | 1.06, 1.05–1.06 | <0.001 | ||
Race | ||||||||
Black/African American versus White | 0.83, 0.78–0.88 | <0.001 | 0.42, 0.36–0.49 | <0.001 | 0.90, 0.79–1.03 | 0.130 | ||
Native Hawaiian and other Pacific Islander versus White | 1.10, 0.89–1.37 | 0.370 | 0.64, 0.44–0.93 | 0.019 | 1.06, 0.67–1.66 | 0.800 | ||
American Indian and Alaska Native versus White | 0.96, 0.70–1.31 | 0.790 | 0.93, 0.55–1.55 | 0.770 | 1.23, 0.67–2.27 | 0.500 | ||
Asian versus White | 1.02, 0.91–1.15 | 0.710 | 0.95, 0.78–1.15 | 0.600 | 0.78, 0.59–1.04 | 0.094 | ||
Others versus White | 1.04, 0.85–1.27 | 0.710 | 0.39, 0.25–0.62 | <0.001 | 0.74, 0.45–1.22 | 0.240 | ||
Unknown versus White | 0.80, 0.69-0.92 | 0.026 | 0.78, 0.56–1.09 | 0.150 | 0.69, 0.47–1.01 | 0.056 | ||
Hispanic origin | ||||||||
Hispanic versus non-Hispanic | 1.07, 0.99–1.16 | 0.088 | 0.72, 0.62–0.83 | <0.001 | 0.77, 0.63–0.93 | 0.061 | ||
Unknown versus non-Hispanic | 0.84, 0.78-0.9 | <0.001 | 0.62, 0.51–0.75 | <0.001 | 0.82, 0.71–0.96 | 0.014 | ||
No high school diploma | ||||||||
10.9–17.5% versus ≥17.6% | 1.06, 1.01–1.11 | 0.012 | 1.22, 1.10–1.36 | 0.025 | 1.14, 1.02–1.26 | 0.015 | ||
6.3–10.8% versus ≥17.6% | 1.12, 1.07–1.17 | <0.001 | 1.52, 1.37–1.68 | <0.001 | 1.18, 1.06–1.30 | 0.018 | ||
<6.3% versus ≥17.6% | 1.23, 1.17–1.29 | <0.001 | 1.92, 1.73–2.13 | <0.001 | 1.09, 0.98–1.22 | 0.100 | ||
Urban/rural | ||||||||
Urban versus metro | 1.11, 1.06–1.16 | <0.001 | 0.92, 0.84–1.02 | 0.110 | 1.24, 1.13–1.36 | <0.001 | ||
Rural versus metro | 1.16, 1.03–1.29 | 0.012 | 0.95, 0.73–1.23 | 0.710 | 1.20, 0.96–1.49 | 0.110 | ||
Charlson/Deyo score | ||||||||
1 versus 0 | 0.88, 0.84–0.91 | <0.001 | 0.75, 0.69–0.82 | <0.001 | 1.32, 1.22–1.42 | <0.001 | ||
2 versus 0 | 0.68, 0.64–0.72 | <0.001 | 0.50, 0.41–0.60 | <0.001 | 1.54, 1.38–1.71 | <0.001 | ||
≥3 versus 0 | 0.57, 0.53–0.61 | <0.001 | 0.35, 0.25–0.47 | <0.001 | 1.75, 1.52–2.01 | <0.001 | ||
Great circle distance | 1.0003, 1.0001–1.0004 | 0.055 | 1.0003, 1.0002–1.0005 | 0.083 | 0.9999, 0.9996–1.0002 | 0.360 |
OR, odds ratio; CI, confidence interval.
Racial and ethnic differences were observed with Blacks having lower odds of receiving both chemotherapy and allogeneic HSCT (chemotherapy OR 0.83, 95% CI: 0.78–0.88; allogeneic HSCT OR 0.42, 95% CI: 0.36–0.49, P<0.001, Table 4) compared to Whites. Hispanics were less likely to receive both allogeneic HSCT and palliative care (allogeneic HSCT OR 0.72, 95% CI: 0.62–0.83, P<0.001; palliative care OR 0.77, 95% CI: 0.63–0.93, P=0.061, Table 4) compared to non-Hispanics. Higher education increased the likelihood of chemotherapy (OR 1.23, 95% CI: 1.17–1.29, P<0.001, Table 4) and allogeneic HSCT (OR 1.92, 95% CI: 1.73–2.13, P<0.001, Table 4). Increasing age (OR 1.06, 95% CI: 1.05–1.06, P<0.001) and Charlson/Deyo scores (score of 1 OR of 1.32, 95% CI: 1.22–1.42; score of 2 OR of 1.54, 95% CI: 1.38–1.71; score of ≥3 OR 1.75, 95% CI: 1.52–2.01; P<0.001 for all) were associated with a higher likelihood of palliative care (Table 4).
Treatment at >1 CoC program was associated with a higher likelihood of palliative care (OR 1.82, 95% CI: 1.13–2.92, P=0.013). In those over age 60, Black patients had a decreased likelihood of chemotherapy and palliative care (chemotherapy OR 0.89, 95% CI: 0.83–0.95, P<0.001; palliative care OR 0.83, 95% CI: 0.71–0.97, P<0.018). However, Hispanics were more likely to receive chemotherapy (OR 1.15, 95% CI: 1.06–1.26, P=0.002) and less likely to receive palliative care (OR 0.75, 95% CI: 0.61–0.93, P=0.007). There were no major differences in receipt of different treatments in our time dependent analyses from 2004–2007, 2008–2011, and 2012–2016.
Discussion
Our study demonstrated differences in TTT, OS, receipt of chemotherapy, allogeneic HSCT and/or palliative care by facility type and volume. In summary, TTT was shorter everywhere when compared to community cancer programs, and at low compared to high volume facilities. Improved OS was seen at academic centers and high volume facilities. Academic centers had a higher likelihood of treatment with chemotherapy and allogeneic HSCT. Palliative care was more likely at low volume facilities. Black patients experienced longer TTT, worse OS and a lower likelihood of treatment with chemotherapy and allogeneic HSCT. Hispanics had longer TTT, but better OS, and were less likely to be treated with allogeneic HSCT and palliative care. There were no differences in our outcomes of interest in time dependent analyses. These results highlight the impact of facility characteristics, demographic, and socioeconomic variables on outcomes in patients with AML, and the need to minimize these discrepancies to optimize patient care.
TTT was shorter at low volume facilities, possibly due to a lower likelihood of using molecular and cytogenetic testing prior to treatment (23). Urgent treatment of AML has historically been favored, but recent data suggests delaying treatment in clinically stable patients while awaiting results of molecular and cytogenetic testing does not have a significant impact on outcomes (24,25). The specific duration to wait without negatively impacting outcomes remains unclear but relates in part to the presence or absence of infection, leukostasis, tumor lysis, or coagulopathy. Sekeres et al. examined rates of complete remission and OS in AML patients ≤60 and >60 years of age, and worse outcomes were observed with longer TTT (≥5 days) among patients in the younger cohort even after adjustments for cytogenetics, risk groups and performance status, but not in patients >60 years (26,27).
Previous studies demonstrated improvements in survival at academic facilities, and NCI designated centers (28-32). Similarly, we saw improved survival at academic centers and high volume facilities. We postulate that there may be greater access to novel treatment options like clinical trials and HSCT, a multi-disciplinary treatment approach with subspecialty services and ancillary staff, and overall greater comfort with managing treatment related complications at such facilities (19,33). AML patients treated with cytarabine-anthracycline induction chemotherapy at hospitals with a high volume of patients receiving induction chemotherapy, were more likely to undergo bone marrow assessments and to receive antibacterial, antifungal and antiviral medications (18).
Historically, patients with hematologic malignancies were less likely to receive palliative care compared to those with solid tumors (34). Integrated palliative care during induction chemotherapy in AML patients improves patient reported and end of life outcomes (35,36). We found a higher likelihood of palliative care services at low volume facilities. This is most likely due to some overlap between low volume facilities, and community cancer centers who frequently care for patients who may favor not pursuing curative therapy, or any therapy all, and thus treatment is classified as palliative care.
Racial and ethnic differences in access to treatments and outcomes in patients with AML persists and are important to understand (6,37-46). Black patients were noted to be less likely to receive allogeneic HSCT to the same extent as White patients (37). Non-Hispanic Black adolescent and young adult (AYA) patients treated on frontline Cancer and Leukemia Group B/Alliance for Clinical Trials in Oncology protocols have higher early death rates, lower rates of complete remission, and lower OS, compared to White patients (47). Clinical trial participation, associated with improved outcomes, was found to be disproportionally higher among Non-Hispanic Whites compared to other racial and ethnic groups (48). We found longer TTT among Blacks and Hispanics, worse OS among Blacks, lower odds of receiving chemotherapy and allogeneic HSCT among Blacks, and lower odds of allogeneic HSCT and palliative care among Hispanics. These poorer outcomes likely reflect the persistent treatment gap and the need to continue to seek equity for these groups. Future studies should focus on gaining insight, perhaps through qualitative work, into factors that influence treatment decisions, and ultimately outcomes in these groups.
Interestingly, Hispanics had better OS compared to non- Hispanics despite the exclusion of acute promyelocytic leukemia, a subtype of AML over-represented among patients of Hispanic origin, and associated with improved outcomes recently due to early recognition and, development of targeted therapies (49). Additionally, in our cohort, Hispanics were diagnosed at a younger age than non-Hispanics, which is consistent with prior work (50). Reasoning behind the observed difference in survival among Hispanics with limited access to treatment options is unknown but has been previously described in the literature as the “Hispanic Mortality Paradox” (51,52). Perhaps the younger age at diagnosis among Hispanics allows for tolerance of more intense treatments. It is worth noting that this survival benefit is not widespread from a geographic location and age perspective. Prior work demonstrates that along the US-Mexico border, in those <35, Hispanic Whites in border regions had greater cancer mortality compared to non-Hispanic Whites in border regions (53). Looking specifically at AML, along the Texas-Mexico border, Hispanics along the border had worse OS than the rest of the state (50). Potential factors described as conferring a survival benefit have included social, psychological, and behavioral differences among Hispanics, and the immigrant phenomenon which confers better outcomes in foreign born compared to US born Hispanics (52,54).
With respect to limitations, our retrospective study design allowed us to make associations but limited our ability to conclude causations and increased the possibility of recall bias. Given the hospital based nature of the NCDB, there was selection bias since we could not elucidate the degree to which patients were referred to specific facilities based on individual clinical characteristics, which ultimately could impact our observed outcomes. With the hospital-based nature of the data, there was no access to patient information for insight into specific treatment decisions including enrollment in clinical trials. Additionally, without molecular/cytogenetic details to stratify risk, we were unable to determine the impact on outcomes. Without disease specific mortality, we could not determine if death was due to AML, related complications, or alternate causes. The 99th percentile was used as our cutoff for high versus low volume facilities since it corresponded to 662 patients, but with establishing this discrete cutoff, we were unable to demonstrate how our outcomes changed progressively with incremental changes in volume. Furthermore, some of these differences, while statistically significant, may not be clinically significant considering the hazard ratio of only slightly greater than 1. Data spanned a wide time frame from 2004–2016, and earlier years in this period may not be representative of the recent advancements in molecular diagnostics and treatment options that have been FDA approved since 2017. Lastly, since the data was derived from the NCDB that includes designated CoC facilities, smaller local facilities that do not qualify for this designation are underrepresented, limiting generalizability to such institutions.
Conclusions
Our study demonstrated significant differences in TTT, OS, and receipt of chemotherapy, allogeneic HSCT, and/or palliative care by facility type and volume. These findings suggest a clinically important discrepancy in care available at academic versus non-academic centers and at low versus high volume facilities. AML largely affects an older population, and care at academic and high volume settings is not always feasible or desirable for these patients. Our data support the need for further understanding of the differences between facility types and development of ways to optimize care at non-academic and low volume facilities. Additionally, there remains important racial and ethnic differences seen among Blacks and Hispanics that require continued efforts to ensure greater access to treatment to all members of the community.
Acknowledgments
The NCDB is a joint project of the Commission on Cancer of the American College of Surgeons and the American Cancer Society. The data used in the study are derived from a de-identified NCDB file. The American College of Surgeons and the Commission on Cancer have not verified and are not responsible for the analytic or statistical methodology employed, or the conclusions drawn from these data by the investigator. We would like to acknowledge Dr. Judith E. Karp for her editorial review of the manuscript. Elements of this work were presented as an oral presentation at the 62nd American Society of Hematology Virtual Annual Meeting on December 6th, 2020.
Funding: None.
Footnote
Reporting Checklist: The authors have completed the STROBE reporting checklist. Available at https://ace.amegroups.com/article/view/10.21037/ace-22-12/rc
Peer Review File: Available at https://ace.amegroups.com/article/view/10.21037/ace-22-12/prf
Conflicts of Interest: All authors have completed the ICMJE uniform disclosure form (available at https://ace.amegroups.com/article/view/10.21037/ace-22-12/coif). KD is on the advisory board for Cellectar for Waldenstroms. CL is on advisory boards for BMS, Jazz Pharma, Genentech, Novartis, Abbvie, Daiichi, Astellas, Macrogenics, Servier, and Taiho; and was on the speakers bureau for Astellas and Jazz. The other authors have no conflicts of interest to declare.
Ethical Statement: The authors are accountable for all aspects of the work in ensuring that questions related to the accuracy or integrity of any part of the work are appropriately investigated and resolved. The study was conducted in accordance with the Declaration of Helsinki (as revised in 2013). This work was exempt from review by the Georgetown University Medical Center Institutional Review Board as the dataset was existing, publicly available, and de-identified.
Open Access Statement: This is an Open Access article distributed in accordance with the Creative Commons Attribution-NonCommercial-NoDerivs 4.0 International License (CC BY-NC-ND 4.0), which permits the non-commercial replication and distribution of the article with the strict proviso that no changes or edits are made and the original work is properly cited (including links to both the formal publication through the relevant DOI and the license). See: https://creativecommons.org/licenses/by-nc-nd/4.0/.
References
- De Kouchkovsky I, Abdul-Hay M. 'Acute myeloid leukemia: a comprehensive review and 2016 update'. Blood Cancer J 2016;6:e441. [Crossref] [PubMed]
- Cancer Stat Facts: Leukemia—Acute Myeloid Leukemia (AML). Available online: https://seer.cancer.gov/statfacts/html/amyl.html
- Bhatnagar B, Kohlschmidt J, Mrózek K, et al. Poor Survival and Differential Impact of Genetic Features of Black Patients with Acute Myeloid Leukemia. Cancer Discov 2021;11:626-37. [Crossref] [PubMed]
- Borate UM, Mineishi S, Costa LJ. Nonbiological factors affecting survival in younger patients with acute myeloid leukemia. Cancer 2015;121:3877-84. [Crossref] [PubMed]
- Jabo B, Morgan JW, Martinez ME, et al. Sociodemographic disparities in chemotherapy and hematopoietic cell transplantation utilization among adult acute lymphoblastic and acute myeloid leukemia patients. PLoS One 2017;12:e0174760. [Crossref] [PubMed]
- Bhatt VR, Shostrom V, Gundabolu K, et al. Utilization of initial chemotherapy for newly diagnosed acute myeloid leukemia in the United States. Blood Adv 2018;2:1277-82. [Crossref] [PubMed]
- Mock J, Meyer C, Mau LW, et al. Barriers to Access to Hematopoietic Cell Transplantation among Patients with Acute Myeloid Leukemia in Virginia. Transplant Cell Ther 2021;27:869.e1-9. [Crossref] [PubMed]
- Bhatt VR, Chen B, Gyawali B, et al. Socioeconomic and health system factors associated with lower utilization of hematopoietic cell transplantation in older patients with acute myeloid leukemia. Bone Marrow Transplant 2018;53:1288-94. [Crossref] [PubMed]
- Joshua TV, Rizzo JD, Zhang MJ, et al. Access to hematopoietic stem cell transplantation: effect of race and sex. Cancer 2010;116:3469-76. [Crossref] [PubMed]
- Bradley CJ, Dahman B, Jin Y, et al. Acute myeloid leukemia: how the uninsured fare. Cancer 2011;117:4772-8. [Crossref] [PubMed]
- Patel MI, Ma Y, Mitchell BS, et al. Understanding disparities in leukemia: a national study. Cancer Causes Control 2012;23:1831-7. [Crossref] [PubMed]
- Master S, Munker R, Shi Z, et al. Insurance Status and Other Non-biological Factors Predict Outcomes in Acute Myelogenous Leukemia: Analysis of Data from the National Cancer Database. Anticancer Res 2016;36:4915-21. [Crossref] [PubMed]
- Byrne MM, Halman LJ, Koniaris LG, et al. Effects of poverty and race on outcomes in acute myeloid leukemia. Am J Clin Oncol 2011;34:297-304. [Crossref] [PubMed]
- Abraham IE, Rauscher GH, Patel AA, et al. Structural racism is a mediator of disparities in acute myeloid leukemia outcomes. Blood 2022;139:2212-26. [Crossref] [PubMed]
- Pulte D, Castro FA, Brenner H, et al. Outcome disparities by insurance type for patients with acute myeloblastic leukemia. Leuk Res 2017;56:75-81. [Crossref] [PubMed]
- Østgård LSG, Nørgaard M, Medeiros BC, et al. Effects of Education and Income on Treatment and Outcome in Patients With Acute Myeloid Leukemia in a Tax-Supported Health Care System: A National Population-Based Cohort Study. J Clin Oncol 2017;35:3678-87. [Crossref] [PubMed]
- Giri S, Pathak R, Aryal MR, et al. Impact of hospital volume on outcomes of patients undergoing chemotherapy for acute myeloid leukemia: a matched cohort study. Blood 2015;125:3359-60. [Crossref] [PubMed]
- Zeidan AM, Podoltsev NA, Wang X, et al. Patterns of care and clinical outcomes with cytarabine-anthracycline induction chemotherapy for AML patients in the United States. Blood Adv 2020;4:1615-23. [Crossref] [PubMed]
- Halpern AB, Walter RB. Practice patterns and outcomes for adults with acute myeloid leukemia receiving care in community vs academic settings. Hematology Am Soc Hematol Educ Program 2020;2020:129-34. [Crossref] [PubMed]
- Levin A, Kleman A, Rein L, et al. Early mortality in patients with acute myelogenous leukemia treated in teaching versus non-teaching hospitals: A large database analysis. Am J Hematol 2017;92:E563-5. [Crossref] [PubMed]
- Deyo RA, Cherkin DC, Ciol MA. Adapting a clinical comorbidity index for use with ICD-9-CM administrative databases. J Clin Epidemiol 1992;45:613-9. [Crossref] [PubMed]
- Venigalla S, Nead KT, Sebro R, et al. Association Between Treatment at High-Volume Facilities and Improved Overall Survival in Soft Tissue Sarcomas. Int J Radiat Oncol Biol Phys 2018;100:1004-15. [Crossref] [PubMed]
- Buccisano F, Maurillo L, Spagnoli A, et al. Cytogenetic and molecular diagnostic characterization combined to postconsolidation minimal residual disease assessment by flow cytometry improves risk stratification in adult acute myeloid leukemia. Blood 2010;116:2295-303. [Crossref] [PubMed]
- Bertoli S, Bérard E, Huguet F, et al. Time from diagnosis to intensive chemotherapy initiation does not adversely impact the outcome of patients with acute myeloid leukemia. Blood 2013;121:2618-26. [Crossref] [PubMed]
- Röllig C, Kramer M, Schliemann C, et al. Does time from diagnosis to treatment affect the prognosis of patients with newly diagnosed acute myeloid leukemia? Blood 2020;136:823-30. [Crossref] [PubMed]
- Sekeres MA, Elson P, Kalaycio ME, et al. Time from diagnosis to treatment initiation predicts survival in younger, but not older, acute myeloid leukemia patients. Blood 2009;113:28-36. [Crossref] [PubMed]
- Kwag D, Cho BS, Min GJ, et al. Effects of Delayed Treatment on Patients with Acute Myeloid Leukemia; Treatment Delay Matters in Younger Patients. Blood 2020;136:19-20. [Crossref]
- Go RS, Bartley AC, Al-Kali A, et al. Effect of the type of treatment facility on the outcome of acute myeloid leukemia in adolescents and young adults. Leukemia 2016;30:1177-80. [Crossref] [PubMed]
- Bhatt VR, Shostrom V, Giri S, et al. Early mortality and overall survival of acute myeloid leukemia based on facility type. Am J Hematol 2017;92:764-71. [Crossref] [PubMed]
- Giri S, Shostrom V, Gundabolu K, et al. Overall Survival (OS) of Acute Myeloid Leukemia (AML) Treated at Academic Center (AC) Versus Non-Academic Center (NAC). Blood 2015;126:533. [Crossref]
- Ho G, Wun T, Muffly L, et al. Decreased early mortality associated with the treatment of acute myeloid leukemia at National Cancer Institute-designated cancer centers in California. Cancer 2018;124:1938-45. [Crossref] [PubMed]
- Law LY, Uong SP, Vempaty HT, et al. Regionalization of Acute Myeloid Leukemia Treatment in a Community-Based Population: Implementation and Early Results. Perm J 2021;25:20.271.
- Hahn AW, Jamy O, Nunnery S, et al. How Center Volumes Affect Early Outcomes in Acute Myeloid Leukemia. Clin Lymphoma Myeloma Leuk 2015;15:646-54. [Crossref] [PubMed]
- LeBlanc TW. In the sandbox: palliative care and hematologic malignancies. J Community Support Oncol 2014;12:44-5. [Crossref] [PubMed]
- El-Jawahri A, LeBlanc TW, Kavanaugh A, et al. Effectiveness of Integrated Palliative and Oncology Care for Patients With Acute Myeloid Leukemia: A Randomized Clinical Trial. JAMA Oncol 2021;7:238-45. [Crossref] [PubMed]
- Nelson AM, Amonoo HL, Kavanaugh AR, et al. Palliative care and coping in patients with acute myeloid leukemia: Mediation analysis of data from a randomized clinical trial. Cancer 2021;127:4702-10. [Crossref] [PubMed]
- Patel MI, Ma Y, Mitchell B, et al. How do differences in treatment impact racial and ethnic disparities in acute myeloid leukemia? Cancer Epidemiol Biomarkers Prev 2015;24:344-9. [Crossref] [PubMed]
- Bierenbaum J, Davidoff AJ, Ning Y, et al. Racial differences in presentation, referral and treatment patterns and survival in adult patients with acute myeloid leukemia: a single-institution experience. Leuk Res 2012;36:140-5. [Crossref] [PubMed]
- Abraham IE, Patel AA, Wang H, et al. Impact of race on outcomes in intermediate-risk acute myeloid leukemia. Cancer Causes Control 2021;32:705-12. [Crossref] [PubMed]
- Darbinyan K, Shastri A, Budhathoki A, et al. Hispanic ethnicity is associated with younger age at presentation but worse survival in acute myeloid leukemia. Blood Adv 2017;1:2120-3. [Crossref] [PubMed]
- Patel MI. Scientific Achievements May Not Reach Everyone: Understanding Disparities in Acute Leukemia. Curr Hematol Malig Rep 2016;11:265-70. [Crossref] [PubMed]
- Pulte D, Redaniel MT, Jansen L, et al. Recent trends in survival of adult patients with acute leukemia: overall improvements, but persistent and partly increasing disparity in survival of patients from minority groups. Haematologica 2013;98:222-9. [Crossref] [PubMed]
- Utuama O, Mukhtar F, Pham YT, et al. Racial/ethnic, age and sex disparities in leukemia survival among adults in the United States during 1973-2014 period. PLoS One 2019;14:e0220864. [Crossref] [PubMed]
- Kirtane K, Lee SJ. Racial and ethnic disparities in hematologic malignancies. Blood 2017;130:1699-705. [Crossref] [PubMed]
- Sekeres MA, Peterson B, Dodge RK, et al. Differences in prognostic factors and outcomes in African Americans and whites with acute myeloid leukemia. Blood 2004;103:4036-42. [Crossref] [PubMed]
- Bhatnagar B, Eisfeld AK. Racial and ethnic survival disparities in patients with haematological malignancies in the USA: time to stop ignoring the numbers. Lancet Haematol 2021;8:e947-54. [Crossref] [PubMed]
- Larkin KT, Nicolet D, Kelly BJ, et al. High early death rates, treatment resistance, and short survival of Black adolescents and young adults with AML. Blood Adv 2022;6:5570-81. [Crossref] [PubMed]
- Hantel A, Luskin MR, Garcia JS, et al. Racial and ethnic enrollment disparities and demographic reporting requirements in acute leukemia clinical trials. Blood Adv 2021;5:4352-60. [Crossref] [PubMed]
- Guru Murthy GS, Szabo A, Michaelis L, et al. Improving Outcomes of Acute Promyelocytic Leukemia in the Current Era: Analysis of the SEER Database. J Natl Compr Canc Netw 2020;18:169-75. [PubMed]
- Bencomo-Alvarez AE, Gonzalez MA, Rubio AJ, et al. Ethnic and border differences on blood cancer presentation and outcomes: A Texas population-based study. Cancer 2021;127:1068-79. [Crossref] [PubMed]
- Durani U, Go RS. Racial and ethnic disparities in the survival of adolescents and young adults with acute myeloid leukemia: a retrospective study using the US National Cancer Data Base. Leuk Lymphoma 2017;58:1184-9. [Crossref] [PubMed]
- Ruiz JM, Steffen P, Smith TB. Hispanic mortality paradox: a systematic review and meta-analysis of the longitudinal literature. Am J Public Health 2013;103:e52-60. [Crossref] [PubMed]
- Paulozzi LJ, McDonald JA, Sroka CJ. A Disparity beneath a Paradox: Cancer Mortality among Young Hispanic Americans in the US-Mexico Border Region. J Racial Ethn Health Disparities 2021;8:1556-62. [Crossref] [PubMed]
- Shor E, Roelfs D, Vang ZM. The "Hispanic mortality paradox" revisited: Meta-analysis and meta-regression of life-course differentials in Latin American and Caribbean immigrants' mortality. Soc Sci Med 2017;186:20-33. [Crossref] [PubMed]
Cite this article as: Taylor AO, Doucette K, Ma X, Chan B, Ahn J, Lai C. Facility type and volume impact outcomes in acute myeloid leukemia—a National Cancer Database Study. Ann Cancer Epidemiol 2023;7:2.