Spatial variation in the incidence of keratinocyte carcinomas at the small area level in Tasmania, Australia: a population-based study
Highlight box
Key findings
• We found strong evidence of spatial variation in keratinocyte carcinoma (KC) incidence at the small area level in the Australian state of Tasmania and stark differences between histological types.
• Basal cell carcinoma (BCC) standardised incidence ratios (SIRs) were highest in the capital city region in the southeast, while squamous cell carcinoma (SCC) SIRs were highest in the northeast and north of the state.
• For BCC, area-level socioeconomic status (SES) contributed to the spatial variation, and SIRs increased with increasing area-level socioeconomic advantage.
What is known and what is new?
• Where we live can influence our risk of being diagnosed with cancer. While KCs are the most common cancers in Australia, their spatial distribution at the small area level by histological type remains unknown.
• A previous study showed differences in BCC incidence by area-level SES, and in SCC incidence by geographic remoteness.
• This study is the first to assess the spatial variation of KCs at the small area level by histological type for an entire Australian state, and to investigate the potential heterogeneity within the broad remoteness and socioeconomic categories previously examined.
What is the implication, and what should change now?
• Spatial differences in sun exposure patterns, ascertainment and treatment modalities could explain the key findings. A better understanding of these likely contributing factors could assist in reducing the burden of these common cancers in Australia.
Introduction
Background
Collectively known as keratinocyte carcinomas (KCs), basal cell carcinoma (BCC) and cutaneous squamous cell carcinoma (SCC) are the commonest cancers in fair-skinned populations (1). In Australia, where incidence is the highest in the world, it has been estimated that 69% of the population will develop a KC in their lifetime (2). Mortality rates are extremely low and KCs account for only 1.2% of all cancer deaths (3). But KCs can cause significant morbidity and, left untreated, they can develop to cause pain, ulceration and disfigurement, substantially impacting quality of life (4). Further, there are significant direct healthcare costs, which in Australia were estimated at $426.2 million in 2021 (5).
It is well established that residential location can influence our risk of a cancer diagnosis (6). Mapping the spatial distribution of cancer can assist in the generation of hypotheses and identification of factors that may be contributing to the rates in a particular area. It can also highlight areas of high risk and provide valuable information to assist in cancer control efforts. For example, maps produced by the Australian Cancer Atlas highlighted the potential for under-diagnosis and under-reporting of myeloproliferative neoplasms in some Australian states (6,7).
Rationale and knowledge gap
Several cancers have been mapped by small area based on cancer registry data (6). But KCs, unlike other neoplasms, are not notifiable diseases and not routinely registered by most Australian population-based cancer registries. Only one Australian study to date has mapped the spatial distribution of KCs by small area. This study was conducted in South Australia using administrative data from Medicare Australia, which does not differentiate between BCC and SCC (8).
Tasmania is the only Australian state, and one of the few jurisdictions globally, that registers BCCs and SCCs. In 2018, BCC and SCC incidence rates in Tasmania were estimated at 891/100,000 and 514/100,000, respectively (9). Our most recent study examined the associations between KC incidence and area-level socioeconomic status (SES) and geographic remoteness in the state and found stark differences by histological type (10). BCC incidence increased with increasing area-level socioeconomic advantage, and SCC incidence was higher in rural than urban areas. However, the study compared estimates for all residents of these areas combined (e.g., all urban areas combined) without considering the potential heterogeneity within these broad remoteness and socioeconomic categories.
Objective
In this study, we aimed to continue our geographical investigation by describing and assessing the spatial distribution and variation in BCC and SCC incidence rates in Tasmania at the small area level. The finer granularity of small area analyses will allow us to understand how patterns differ within the broader geographic categories studied previously. We present this article in accordance with the STROBE reporting checklist (available at https://ace.amegroups.com/article/view/10.21037/ace-24-22/rc).
Methods
Study design and population
This population-based study was conducted in Tasmania, the southernmost Australian state. It has a predominantly Anglo-Saxon population of approximately 570,000 people and a median age of 45 years, the oldest in Australia (11,12). Tasmania’s largest cities are Hobart (the capital city) in the southeast, followed by Launceston and Burnie in the north.
De-identified records of Tasmanian residents registered with histologically confirmed BCCs and SCCs between 1 January 2014 and 31 December 2018 by the Tasmanian Cancer Registry were obtained. Registry data date back to 1978, but our previous study indicated no substantial changes in the distribution of incidence rates across different socioeconomic and remoteness areas over time; therefore, our study seeks to examine only recent spatial patterns. Further, small area classifications change over time, hindering valid temporal comparisons. Detailed information on the registration and coding practices of KCs by the Registry has been described previously (9). In brief, the Tasmanian Cancer Registry is a population-based registry covering the whole of Tasmania. Histologically confirmed KCs are notified to the Registry by pathology laboratories and public and private hospitals. Lesions with a skin site (C44), morphology codes 805–808 or 809–811, and behaviour code 3 (malignant, primary) were respectively ascertained from pathology reports as SCCs or BCCs according to the International Classification of Diseases for Oncology, Third Edition (13). Records belonging to the same individuals were identified using probabilistic data linkage. Only the first cancer diagnosis of each histological type was registered per person per year of diagnosis, regardless of the body subsite, and recurrent lesions, where identified in the pathology report, were excluded.
The person’s residential address at diagnosis was geocoded and assigned to a Statistical Area level 2 (SA2) according to the 2016 Australian Statistical Geography Standard (14). During 2014–2018, the address was missing for 42 BCCs and 69 SCCs, and these were excluded from the analysis. SA2s represent medium-sized communities that interact socially and economically and are generally the smallest areas for which population data are available. Data on the estimated resident population, area-level SES and geographic remoteness were sourced from the Australian Bureau of Statistics (15-17).
This study was approved by the Tasmanian Health and Medical Human Research Ethics Committee (reference H0018089) and was conducted in accordance with the principles outlined in the National Statement on Ethical Conduct in Human Research 2007 (updated 2018), as published by the National Health and Medical Research Council, and the Declaration of Helsinki (as revised in 2013). It was not possible to obtain informed consent because many subjects are deceased, and data are for an entire population.
Outcomes
The outcome was the total number of persons diagnosed with a lesion during 2014–2018, with a maximum of one new lesion per person, histological type and calendar year. To ensure that the variance estimates at the small area were valid, we used the total counts over the five years combined, but results are reported as the average counts of persons diagnosed per calendar year. Indirectly standardised incidence ratios (SIRs) with 95% credible intervals (CrIs) and counts were used to report incidence at the small area. Incidence rates, standardised to the Australian 2001 population (11), were used to describe incidence for all areas combined.
Standardisation removes the effect of differences in the age structure and population size in different areas. In small area statistics, indirect age-standardisation is preferred over direct age-standardisation because it reduces the sampling variation that arises from the number of cases by age group (18,19). This method is also advantageous when comparing rates from a large number of subpopulations because they provide insight into the rates relative to the baseline or study average. The SIR compares the incidence rate in a small area with that of the whole Tasmanian population and represents the ratio of the number of cases to the number of expected cases if the age-specific incidence rates were constant across the study area. An SIR greater than 1.00 suggests that the number of persons diagnosed was higher than expected; an SIR lower than 1.00 suggests that the number of persons diagnosed was lower than expected. The exceedance probabilities were calculated as per Duncan and colleagues (18) to provide evidence whether a local area’s rates were considered truly different to the Tasmanian rate. Areas with an exceedance probability less than 0.2 or higher than 0.8 were considered to have strong evidence that rates were truly lower or higher (respectively) than the Tasmanian rate (20).
Covariates
Area-level SES and geographic remoteness were assessed individually as covariates within the spatial models because they are associated with KC incidence in Tasmania (10) and may, therefore, contribute to spatial patterns. Area-level SES was ranked into five categories based on quintiles of the Tasmanian SA2s’ scores for the 2016 Index of Relative Socio-economic Advantage and Disadvantage (IRSAD) (16). Geographic remoteness was categorised as “urban” (i.e., “inner regional”) vs. “rural” (“outer regional”, “remote” and “very remote”) according to the Remoteness Structure classification (Tasmania does not have a “major city”) (17).
Statistical analysis
Analysis was conducted at the SA2 level. Tasmania has 99 SA2s, but areas with an average annual population of less than 50 persons were excluded from the analysis (n=3; no KCs were registered in these areas).
Small area statistics are subject to sampling variability due to the small number of cases in each area. Modelling the number of cases over a five-year-period addresses some of the random variation. Spatial smoothing, which utilises information from neighbouring areas to estimate the incidence rate, further reduces the sampling variability to reveal the latent patterns beneath the random fluctuations in the observed data. Spatial smoothing also protects confidentiality, provides insight into the uncertainty in the estimates and accounts for the correlation between neighbouring areas (18).
Following recommendations from Cramb and colleagues, we used Bayesian spatial models to apply spatial smoothing, and specifically the conditional autoregressive model (21). The Besag, York and Mollié (BYM) model (22) was selected because it offered the best model fit. Details of the BYM model have been described previously (21). In brief, Bayesian models account for spatial autocorrelation (i.e., the tendency for areas that are nearby to have similar characteristics) by considering neighbouring values when estimating the probabilities for an area. Bayesian spatial models require a spatial weights matrix, which has been specified as a binary adjacency matrix of neighbouring areas. Areas were neighbours if they shared a boundary, and in the case of Tasmania’s two island SA2s (i.e., “King Island” and “Flinders and Cape Barren Islands”), the closest SA2s based on straight line distance were assigned as neighbours.
Analysis was conducted in R version 4.1.0 (23). Small area incidence data were modelled using CARBayes (version 5.3) (24). Models were built and outcomes were mapped separately for BCC and SCC for males, females and persons, as previous studies suggested differences by histological type and sex (9,10). Each model was then adjusted separately for area-level SES and geographic remoteness to investigate their spatial effects. Model convergence was assessed by visually examining trace plots and using Geweke diagnostics, where a P value greater than 0.05 indicated within-chain convergence. Three chains were run each with different initial values and assessed using the Gelman-Rubin convergence diagnostic, for which upper limits less than 1.05 indicated between-chain convergence. Moran’s I test assessed evidence of spatial autocorrelation in the model residuals. The models’ fit was assessed using the Deviance Information Criterion (DIC), the Watanabe-Akaike Information Criterion (WAIC) and the Log Marginal Predictive Likelihood (LMPL). To determine the relative risk (RR) for the categorical covariates, we took the median and 95% CrIs of the regression coefficients. A sensitivity analysis indicated that results were independent of the initial values and choice of priors.
Tango’s Maximised Excess Events Test (MEET) global clustering test (25) assessed the evidence for spatial variation in modelled SIRs. In this test, the modelled counts (i.e., the product of the modelled SIR and the number of expected cases) were compared with the expected counts in small areas.
Results
A total of 96 areas were included in the analysis. Among these areas, 63% were classified as urban (n=60) and 38% were classified as rural (n=36). An average of 6,566 persons were registered with BCCs (40% females) and 3,864 were registered with SCCs (40% females) each year during 2014–2018, representing an average age-standardised incidence rate of 932/100,000 and 525/100,000, respectively. The outcomes of the spatial analyses were similar for males and females; therefore, the results focus on all persons combined.
SIRs
There was strong evidence of spatial variation for both histological types (Tango’s MEET P value <0.001). Models for both BCC and SCC had a Moran’s I P value of >0.05, indicating no evidence of spatial autocorrelation in the residuals and adequate smoothing by the models.
For BCC, incidence was statistically significantly higher than expected in 23% of areas (n=22), and lower than expected in 34% of areas (n=34). Most of the areas with high SIR were in the southeast and east of the state, especially in the capital city of Hobart that is located in the southeast. Out of the 34 areas that compose the capital city, 47% (n=16) had incidence that was statistically significantly higher than expected (e.g., Howrah-Tranmere, SIR 1.49, 95% CI: 1.40, 1.58; Bellerive-Rosny, SIR 1.44, 95% CI: 1.33, 1.55) (Figure 1).
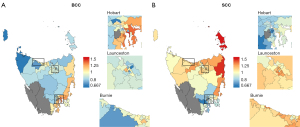
For SCC, incidence was statistically significantly higher than expected in 25% of areas (n=24), and lower than expected in 28% of areas (n=27). Incidence was highest in the north and northeast coast of the state (e.g., Flinders and Cape Barren Islands, SIR 1.78, 95% CI: 1.46, 2.17; St Helens-Scamander, SIR 1.48 95% CI: 1.35, 1.61). The lowest SIRs were all in the southeast of the state, including in Hobart, where 68% (n=23) of areas had incidence that was lower than expected (Figure 1). These spatial patterns were mostly similar for both sexes (Figure 2).
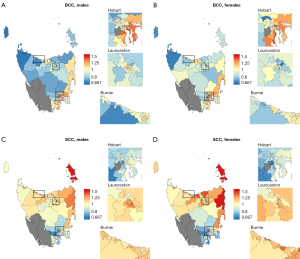
Among areas with statistically significant SIRS, five percent (n=5) had consistently high SIRs and 9% (n=9) had consistently low SIRs across both histological types. Out of the areas with consistently low SIRs, two thirds (n=6) were in the northern suburbs of the city of Hobart. Areas with consistently high SIRs were spread across the state in the cities of Hobart and Launceston, and the east coast (Figure 1).
The effect of area-level SES and geographic remoteness
For BCC, inclusion of area-level SES improved model fit. This was evidenced by a reduction in the DIC (e.g., DIC: 898.14 with covariate; 905.32 without covariate), a reduction in WAIC (WAIC: 883.99 with covariate; 888.63 without covariate) and an increase in LMPL (LMPL: −479.58 with covariate; −491.63 without covariate) (Table 1).
Table 1
Model† by sex | BCC | SCC | |||||
---|---|---|---|---|---|---|---|
DIC | WAIC | LMPL | DIC | WAIC | LMPL | ||
Males | |||||||
Unadjusted | 851.08 | 838.27 | −465.40 | 778.85§ | 772.64§ | −402.93§ | |
Adjusted for geographic remoteness‡ | 851.45 | 838.61 | −463.51 | 779.91 | 773.61 | −405.82 | |
Adjusted for area-level SES | 844.02§ | 833.10§ | −449.47§ | 780.26 | 773.62 | −406.60 | |
Females | |||||||
Unadjusted | 808.10 | 801.54 | −434.31 | 742.22§ | 738.41§ | −395.61§ | |
Adjusted for geographic remoteness‡ | 808.18 | 801.34 | −432.31 | 742.43 | 739.59 | −395.83 | |
Adjusted for area-level SES | 798.68§ | 793.99§ | −420.91§ | 743.40 | 738.46 | −393.46 | |
Persons | |||||||
Unadjusted | 905.32 | 888.63 | −491.63 | 843.97§ | 833.79§ | −455.48§ | |
Adjusted for geographic remoteness‡ | 906.40 | 890.01 | −500.92 | 844.81 | 834.83 | −454.82 | |
Adjusted for area-level SES | 898.14§ | 883.99§ | −479.58§ | 844.51 | 833.03 | −446.53 |
†, Bayesian spatial models used to smooth standardised incidence ratios; ‡, urban vs. rural; §, the model with the lowest DIC and WAIC and highest LMPL had the best fit. BCC, basal call carcinoma; DIC, Deviance Information Criterion; LMPL, Log Marginal Predictive Likelihood; SCC, squamous cell carcinoma; SES, socioeconomic status; WAIC, Watanabe-Akaike Information Criterion.
The small area-specific SIRs for BCC generally increased with increasing area-level SES (Figure 3). Compared with areas of most socioeconomic disadvantage (category 1), the RR of a BCC diagnosis was 22% higher in areas of socioeconomic advantage (category 4; RR 1.22, 95% CrI: 1.11, 1.34) and 47% higher in areas of most socioeconomic advantage (category 5; RR 1.47, 95% CrI: 1.33, 1.63) (Table 2). Exceedance probabilities provided strong evidence that 95% (n=18) of the areas categorized as most socioeconomically advantaged had strong statistically significant evidence of high SIRs, and 79% (n=15) of the areas categorized as most socioeconomically disadvantaged had strong statistically significant evidence of low SIRs (Table 3). No areas categorized as “most disadvantaged” had higher-than-expected incidence rates and no areas categorized as “most advantaged” had lower-than-expected incidence rates.
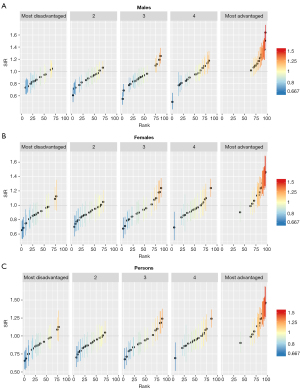
Table 2
Area-level SES | RR | 95% CrI |
---|---|---|
1 (most disadvantaged) | 1.00 | Ref. |
2 | 1.06 | 0.97, 1.17 |
3 | 1.10 | 1.01, 1.21 |
4 | 1.22 | 1.11, 1.34 |
5 (most advantaged) | 1.47 | 1.33, 1.63 |
Area-level SES categories are ranked from 1 (most disadvantaged) to 5 (most advantaged). CrI, credible interval; Ref., reference; RR, relative risk; SES, socioeconomic status.
Table 3
Exceedance probability | Area-level SES, % [n] | ||||
---|---|---|---|---|---|
1 (most disadvantaged) | 2 | 3 | 4 | 5 (most advantaged) | |
Low | 79% [15] | 74% [14] | 80% [16] | 53% [10] | 0% [0] |
Unclear | 21% [4] | 21% [4] | 0% [0] | 21% [4] | 5% [1] |
High | 0% [0] | 5% [1] | 20% [4] | 26% [5] | 95% [18] |
Area-level SES categories are ranked from 1 (most disadvantaged) to 5 (most advantaged). Low: lower than the Tasmanian average; Unclear: unclear if different to the Tasmanian average; High: higher than the Tasmanian average. SES, socioeconomic status.
For BCC, the inclusion of geographic remoteness as a covariate did not improve the model fit. For SCC, inclusion of neither area-level SES nor geographic remoteness improved the model fit (Table 1).
Discussion
Key findings
This was the first population-based Australian study to examine the spatial distribution of BCC and SCC incidence across small areas in the state of Tasmania. We found strong evidence of spatial variation and stark contrasts in the spatial patterns between the two histological types. BCC incidence was highest in the capital city region in the southeast, while SCC incidence was highest in the northeast and north of the state. For BCC, area-level SES contributed to the spatial variation, and incidence increased with increasing area-level socioeconomic advantage.
Strengths and limitations
This study has strengths. First, it uses novel population level, cancer registry-based data, which has allowed us to visualise, for the first time, the spatial patterns of KC incidence by histological type for Tasmania at the small area level. Second, our study uses Bayesian spatial hierarchical models, which provide area-level smoothing to minimise the impact of chance variation that could have led to erroneous conclusions. This study also has limitations. First, caution is needed when inferring the risk of individuals living in an area from aggregate risk for the area. This is because area-level risks cannot be used to infer the individual risk for persons living in that area (i.e., ecological fallacy). Second, lesions that were not sent for histological confirmation, such as those treated using destructive therapies, are not collected by the Tasmanian Cancer Registry and, therefore, could not be included in this analysis.
Comparison with similar researches
For BCC, incidence rates were highest in the southeast, especially in the capital city of Hobart. Geographic remoteness did not explain the variation because the small-area spatial patterns were more nuanced than these broad geographic categories could describe. Nonetheless, our findings largely support the only other comparable population-based cancer registry spatial analysis from Ireland that found higher BCC incidence in urban areas (26). A recent study from Wales that used linked cancer registry and healthcare data also reported higher BCC incidence in urban areas (27); however, the study did not use spatial modelling, which hinders a direct comparison. Although there are differences in climate and latitude between Tasmania, Ireland and Wales, at the local level service availability and accessibility disparities between larger cities and more regional areas likely play a more influential role in small area variations. Further, consistent with our previous study and several other studies (10,26-33), BCC incidence increased with increasing area-level SES. Hobart has the highest concentration of socioeconomically advantaged areas, especially in the southern and eastern suburbs, and this contributed to the higher incidence rates observed in the region.
For SCC, incidence rates were highest in the north and northeast and lowest in the southeast of the state. This is also partly consistent with the Irish study that found that SCC was more evenly spread across the country and less concentrated in the larger cities than BCC (26). Our previous study found that SCC incidence was higher among those living in rural than urban areas (10), but in our current study geographic remoteness did not explain the spatial variation, appearing to contradict our previous finding. However, this might be explained by differences in the unit of analysis between the two studies. In our previous study, the geographical unit of analysis was two broad regions (i.e., urban and rural). In the current study, the unit of analysis was the small area (n=96 small areas covering Tasmania). This could indicate heterogeneity in SCC rates among areas categorised as “urban”. For instance, small areas in the broader regions of Launceston and Burnie are all classified as urban, as in the broader region of Hobart, but the small area analyses revealed very different patterns between these different regions.
Explanations of findings
Exposure to ultraviolet (UV) radiation from the sun is the largest risk factor for skin cancers (34) and different patterns of sun exposure have been associated with the development of different histological types. Some evidence suggests that BCCs develop in association with more intermittent sun exposure, while the development of SCC is associated with more continuous and cumulative sun exposure (35). UV levels in Tasmania are lower than in mainland Australia and spatial variations across the island are only marginal (36). But differences in the distribution of sun exposure patterns could explain the findings. Residents of areas of high SES in the city tend to occupy white-collar jobs (37) that potentially lend themselves to more intermittent sun exposure patterns, where weekdays are spent in the office and leisure time is spent outdoors, including holidays to sunny locations closer to the equator where UV levels are higher. In addition, historical trends in tanning behaviour could be a factor. Tanning became fashionable in the 1920s and 1930s and the decades that followed saw the emergence of UV reflectors, tanning oils and eventually solaria (which emit high levels of UV radiation) (38,39), all of which might have been more available to those who were more socioeconomically advantaged and had more leisure time and resources to pursue a tan. Cancer develops as the result of an accumulation of genetic changes over time, potentially over many decades of exposure (40). The elevated BCC incidence in areas of socioeconomic advantage could reflect current and past trends in tanning behaviour, but further investigations are needed to confirm this.
For SCC, it is possible that those living outside the capital city experience more continuous patterns of sun exposure due to more outdoor lifestyles and occupations. For instance, regional Tasmania has a higher percentage of persons employed in Agriculture, Forestry and Fishing than the greater Hobart area (8% and 2%, respectively) (41). This includes the city of Launceston in the north, which, despite being an urban area, is considered a major agricultural and pastoral hub. Australian farmers are more frequently exposed to UV radiation than other outdoor workers and have been reported to display suboptimal skin protective behaviours. For instance, in the southern Australian state of Victoria, farmers spent the most time outdoors among outdoor workers (i.e., 6 days compared with 5 days for other outdoor workers), and while most farmers reported usually/always wearing a hat (78%), 22% did not and a further 78% reported not usually/always applying sunscreen (42). A recent mixed methods study identified barriers and solutions to sun protection among Australian farmers and highlighted the need for tailored health promotion messages to target this population (43). Health policy makers should consider this when developing and implementing sun awareness campaigns in Tasmania and Australia more broadly.
Ascertainment differences could also partly explain the findings. Most KCs develop slowly and rarely metastasise and cause death. The increasing awareness of skin cancer in Australia over the past decades due to awareness campaigns contributed to increased surveillance and therefore detection of low-risk and asymptomatic skin lesions (44). This trend was likely magnified by the emergence of primary care skin cancer clinics across Australian cities since the early 2000s (45). The detection of low-risk and asymptomatic KCs can often lead to overdiagnosis—that is, the diagnosis and treatment of lesions that would have never developed to cause harm within one’s lifetime. Both BCCs and SCCs are candidates for overdiagnosis, but BCCs are more indolent than SCCs and have been tagged as a particularly strong candidate in this respect (46). The capital city of Hobart has both the highest BCC incidence and the highest concentration of general practitioner clinics, skin cancer clinics and hospitals in the state. Regular skin checks are recommended to specific high-risk groups (47) but it is unclear whether this translates to clinical practice in an equitable way. And while it is unknown how many skin cancers are incidentally diagnosed during other routine health checks, it is likely that a higher number of these incidental findings are detected in areas where there are fewer barriers to health services (e.g., higher levels of availability, accessibility and affordability). Further, higher levels of health literacy among those of higher SES (48) could result in higher levels of awareness of skin cancer and therefore higher detection rates. Conversely, underdiagnosis could also be a factor in areas where there are more barriers to health services and lower levels of health literacy.
There is the possibility of area-level differences in treatment modalities. Surgical excision is the gold standard treatment for KCs, and histological confirmation is a prerequisite for items to be billed by primary care practitioners so that they can be subsidised by Medicare Australia (49). Non-surgical treatment modalities, such as cryotherapy, can be performed without histological confirmation, in which case they are not reported to the Tasmanian Cancer Registry. The number of non-surgical treatments performed for KCs without histological confirmation is unknown because there is no specific billing code under Medicare Australia. It is also unknown whether treatment modalities vary across Tasmania, but it seems unlikely that these played a role in producing the broader spatial patterns we observed.
Implications and actions needed
Area-level SES explained some of the spatial variation for BCC, but the role of other contributing factors is uncertain. Future research could examine the influence of other likely factors, such as spatial variations in (I) the distributions of risk factors for KC, (II) skin cancer awareness, (III) under- or overdiagnosis, (IV) clinical practice and (V) the detection and reporting of KCs due to differing levels of service availability, accessibility and affordability.
Conclusions
Our study found high levels of spatial variation in KC incidence at the small area level in Tasmania, Australia, and stark contrasts between the two histological types. For BCC, area level SES contributed to the observed spatial patterns, but other contributing factors are uncertain. KCs are a substantial burden in Australia and fair-skinned populations globally, and a better understanding of these factors could assist efforts to control these common cancers.
Acknowledgments
The authors thank the staff of the Tasmanian Cancer Registry for the collection of data and coding advice, the staff of the Tasmanian Data Linkage Unit for the data linkage, and the Australian Cancer Atlas team for kindly providing the RStudio code for the analysis.
Footnote
Reporting Checklist: The authors have completed the STROBE reporting checklist. Available at https://ace.amegroups.com/article/view/10.21037/ace-24-22/rc
Data Sharing Statement: Available at https://ace.amegroups.com/article/view/10.21037/ace-24-22/dss
Peer Review File: Available at https://ace.amegroups.com/article/view/10.21037/ace-24-22/prf
Funding: The article was supported in part by
Conflicts of Interest: All authors have completed the ICMJE uniform disclosure form (available at https://ace.amegroups.com/article/view/10.21037/ace-24-22/coif). P.B. serves as an unpaid editorial board member of Annals of Cancer Epidemiology from Jan 2024 to Dec 2025. J.R. reports consultancies with University of Birmingham Enterprise, outside the submitted work. The other authors have no conflicts of interest to declare.
Ethical Statement: The authors are accountable for all aspects of the work in ensuring that questions related to the accuracy or integrity of any part of the work are appropriately investigated and resolved. The study was conducted in accordance with the principles outlined in the National Statement on Ethical Conduct in Human Research 2007 (Updated 2018), as published by the National Health and Medical Research Council, and the Declaration of Helsinki (as revised in 2013). The study was approved by the Tasmanian Health and Medical Human Research Ethics Committee (reference H0018089). It was not possible to obtain informed consent because many subjects are deceased, and data are for an entire population.
Open Access Statement: This is an Open Access article distributed in accordance with the Creative Commons Attribution-NonCommercial-NoDerivs 4.0 International License (CC BY-NC-ND 4.0), which permits the non-commercial replication and distribution of the article with the strict proviso that no changes or edits are made and the original work is properly cited (including links to both the formal publication through the relevant DOI and the license). See: https://creativecommons.org/licenses/by-nc-nd/4.0/.
References
- Lomas A, Leonardi-Bee J, Bath-Hextall F. A systematic review of worldwide incidence of nonmelanoma skin cancer. Br J Dermatol 2012;166:1069-80. [Crossref] [PubMed]
- Olsen CM, Pandeya N, Green AC, et al. Keratinocyte cancer incidence in Australia: a review of population-based incidence trends and estimates of lifetime risk. Public Health Res Pract 2022;32:3212203. [Crossref] [PubMed]
- Australian Institute of Health and Welfare. Skin cancer in Australia. Canberra: AIHW; 2016.
- Gaulin C, Sebaratnam DF, Fernández-Peñas P. Quality of life in non-melanoma skin cancer. Australas J Dermatol 2015;56:70-6. [Crossref] [PubMed]
- Gordon LG, Leung W, Johns R, et al. Estimated Healthcare Costs of Melanoma and Keratinocyte Skin Cancers in Australia and Aotearoa New Zealand in 2021. Int J Environ Res Public Health 2022;19:3178. [Crossref] [PubMed]
- Cancer Council Queensland, Queenland University of Technology, Cooperative Research Centre for Spatial Information. Australian Cancer Atlas. Version 02-2021. Available online: https://atlas.cancer.org.au [accessed 6 May 2023].
- Cameron JK, Fritschi L, Ross DM, et al. Spatial disparities in the reported incidence and survival of myeloproliferative neoplasms in Australia. Pathology 2022;54:328-35. [Crossref] [PubMed]
- Adelson P, Sharplin GR, Roder DM, et al. Keratinocyte cancers in South Australia: incidence, geographical variability and service trends. Aust N Z J Public Health 2018;42:329-33. [Crossref] [PubMed]
- Ragaini BS, Blizzard L, Newman L, et al. Temporal trends in the incidence rates of keratinocyte carcinomas from 1978 to 2018 in Tasmania, Australia: a population-based study. Discov Oncol 2021;12:30. [Crossref] [PubMed]
- Ragaini BS, Blizzard L, Baade P, et al. Keratinocyte carcinomas, area-level socioeconomic status and geographic remoteness in Tasmania: cross-sectional associations and temporal trends. Aust N Z J Public Health 2023;47:100067. [Crossref] [PubMed]
- Australian Bureau of Statistics. National, state and territory population: Australian Bureau of Statistics; 2023. Available online: https://www.abs.gov.au/statistics/people/population/national-state-and-territory-population/latest-release#states-and-territories [accessed 06 October 2023].
- Australian Bureau of Statistics. Regional population by age and sex: Australian Bureau of Statistics; 2023. Available online: https://www.abs.gov.au/statistics/people/population/regional-population-age-and-sex/latest-release#tasmania [accessed 06 October 2023].
- Fritz A, Percy C, Jack A, et al. International Classification of Diseases for Oncology. 3rd edition. World Health Organization; 2013.
- Australian Bureau of Statistics. Australian Statistical Geography Standard (ASGS): Volume 1 - Main Structure and Greater Capital City Statistical Areas, July 2016. Cat no. 1270.0.55.001. Canberra; 2016.
- Australian Bureau of Statistics. Regional population: Australian Bureau of Statistics; 2023. Available online: https://www.abs.gov.au/statistics/people/population/regional-population/latest-release [accessed 06 October 2023].
- Australian Bureau of Statistics. Census of Population and Housing: Socio-Economic Indexes for Areas (SEIFA), Australia, 2016. Canberra: Australian Bureau of Statistics; 2018.
- Australian Bureau of Statistics. Australian Statistical Geography Standard (ASGS): Volume 5 - Remoteness Structure, July 2016. Canberra: Australian Bureau of Statistics; 2018.
- Duncan EW, Cramb SM, Aitken JF, et al. Development of the Australian Cancer Atlas: spatial modelling, visualisation, and reporting of estimates. Int J Health Geogr 2019;18:21. [Crossref] [PubMed]
- Pickle LW. A history and critique of U.S. mortality atlases. Spat Spatiotemporal Epidemiol 2009;1:3-17. [Crossref] [PubMed]
- Richardson S, Thomson A, Best N, et al. Interpreting posterior relative risk estimates in disease-mapping studies. Environ Health Perspect 2004;112:1016-25. [Crossref] [PubMed]
- Cramb S, Duncan E, Baade P, et al. Investigation of Bayesian spatial models. Brisbane: Cancer Council Queensland and Queensland University of Technology (QUT); 2018. Available online: https://eprints.qut.edu.au/115590/
- Besag J, York J, Mollié A. Bayesian image restoration, with two applications in spatial statistics. Ann Inst Stat Math 1991;43:1-20. [Crossref]
- R Core Team. R: A language and environment for statistical computing Vienna, Austria. 2021. Available online: https://www.R-project.org/
- Lee D. CARBayes: an R package for Bayesian spatial modeling with conditional autoregressive priors. J Stat Softw 2013;1-24. [Crossref]
- Tango T. A test for spatial disease clustering adjusted for multiple testing. Stat Med 2000;19:191-204. [Crossref] [PubMed]
- Carsin AE, Sharp L, Comber H. Geographical, urban/rural and socioeconomic variations in nonmelanoma skin cancer incidence: a population-based study in Ireland. Br J Dermatol 2011;164:822-9. [Crossref] [PubMed]
- Ibrahim N, Jovic M, Ali S, et al. The epidemiology, healthcare and societal burden of basal cell carcinoma in Wales 2000-2018: a retrospective nationwide analysis. Br J Dermatol 2023;188:380-9. [Crossref] [PubMed]
- Deady S, Sharp L, Comber H. Increasing skin cancer incidence in young, affluent, urban populations: a challenge for prevention. Br J Dermatol 2014;171:324-31. [Crossref] [PubMed]
- Aarts MJ, van der Aa MA, Coebergh JW, et al. Reduction of socioeconomic inequality in cancer incidence in the South of the Netherlands during 1996-2008. Eur J Cancer 2010;46:2633-46. [Crossref] [PubMed]
- Corazza M, Ferretti S, Scuderi V, et al. Socioeconomic status and skin cancer incidence: a population-based, cohort study in the province of Ferrara, northern Italy. Clin Exp Dermatol 2021;46:1285-9. [Crossref] [PubMed]
- Doherty VR, Brewster DH, Jensen S, et al. Trends in skin cancer incidence by socioeconomic position in Scotland, 1978-2004. Br J Cancer 2010;102:1661-4. [Crossref] [PubMed]
- van Hattem S, Aarts MJ, Louwman WJ, et al. Increase in basal cell carcinoma incidence steepest in individuals with high socioeconomic status: results of a cancer registry study in The Netherlands. Br J Dermatol 2009;161:840-5. [Crossref] [PubMed]
- Ray GT, Kulldorff M, Asgari MM. Geographic Clusters of Basal Cell Carcinoma in a Northern California Health Plan Population. JAMA Dermatol 2016;152:1218-24. [Crossref] [PubMed]
- International Agency for Research on Cancer. IARC monographs on the evaluation of carcinogenic risks to humans: solar and ultraviolet radiation. Volume 55. Lyon, France: World Health Organization; 1992.
- Rosso S, Zanetti R, Martinez C, et al. The multicentre south European study 'Helios'. II: Different sun exposure patterns in the aetiology of basal cell and squamous cell carcinomas of the skin. Br J Cancer 1996;73:1447-54. [Crossref] [PubMed]
- Australian Government Bureau of Meteorology. Average solar ultraviolet (UV) Index 2023. Available online: http://www.bom.gov.au/jsp/ncc/climate_averages/uv-index/index.jsp?period=an#maps [accessed 07 May 2023].
- Australian Bureau of Statistics. 2016 Census of Population and Housing. TableBuilder. Findings based on use of ABS TableBuilder data. Canberra: Australian Bureau of Statistics; 2016.
- Makin JK, Dobbinson SJ, Herd NL. The increase in solariums in Australia, 1992-2006. Aust N Z J Public Health 2007;31:191-2. [Crossref] [PubMed]
- Aldahan AS, Shah VV, Mlacker S, et al. Sun Exposure in History. JAMA Dermatol 2016;152:896. [Crossref] [PubMed]
- Cooper GM. The development and causes of cancer. The cell: a molecular approach. 2nd edition. Sunderland (MA): Sinauer Associates; 2000:719-28.
- Australian Bureau of Statistics. 2021 Census of Population and Housing. TableBuilder. Findings based on use of ABS TableBuilder data. Canberra: Australian Bureau of Statistics; 2021.
- Dobbinson S, Doyle C, Wakefield M. Farmers’ and outdoor workers’ belief about skin cancer and protection from summer sun: a brief report. CBRC Research Paper Series. No. 18. 2005. Available online: https://www.cancervic.org.au/research/behavioural/research-papers/abstracts_beliefs_on_skin_canc.html#:~:text=CBRC%20Research%20Paper%20Series%20No,18&text=Over%2083%25%20of%20farmers%20and,cancer%20each%20year%20in%20Australia
- Trenerry C, Fletcher C, Wilson C, et al. "She'll Be Right, Mate": A Mixed Methods Analysis of Skin Cancer Prevention Practices among Australian Farmers-An At-Risk Group. Int J Environ Res Public Health 2022;19:2940. [Crossref] [PubMed]
- Iannacone MR, Green AC. Towards skin cancer prevention and early detection: evolution of skin cancer awareness campaigns in Australia. Melanoma Manag 2014;1:75-84. [Crossref] [PubMed]
- Wilkinson D, Dick ML, Askew DA. General practitioners with special interests: risk of a good thing becoming bad? Med J Aust 2005;183:84-6. [Crossref] [PubMed]
- Linos E, Schroeder SA, Chren MM. Potential overdiagnosis of basal cell carcinoma in older patients with limited life expectancy. JAMA 2014;312:997-8. [Crossref] [PubMed]
- Cancer Council Australia Keratinocyte Cancers Guideline Working Party. Clinical practice guidelines for keratinocyte cancer. 2019. Available online: https://wiki.cancer.org.au/australia/Guidelines:Keratinocyte_carcinoma [accessed 10 August 2023].
- Beauchamp A, Buchbinder R, Dodson S, et al. Distribution of health literacy strengths and weaknesses across socio-demographic groups: a cross-sectional survey using the Health Literacy Questionnaire (HLQ). BMC Public Health 2015;15:678. [Crossref] [PubMed]
- Medicare Australia. Education guide - Billing skin lesion treatment and biopsy items under Medicare 2023. Available online: https://www.servicesaustralia.gov.au/organisations/health-professionals/topics/education-guide-billing-skin-lesion-treatment-and-biopsy-items-under-medicare/33226 [accessed 23 September 2023].
Cite this article as: Ragaini BS, Cameron J, Baade P, Roydhouse J, Blizzard L, Venn A. Spatial variation in the incidence of keratinocyte carcinomas at the small area level in Tasmania, Australia: a population-based study. Ann Cancer Epidemiol 2025;9:1.